The Latest Buzz
How Do Perception Models Help Robotaxis Navigate Complex Environments?
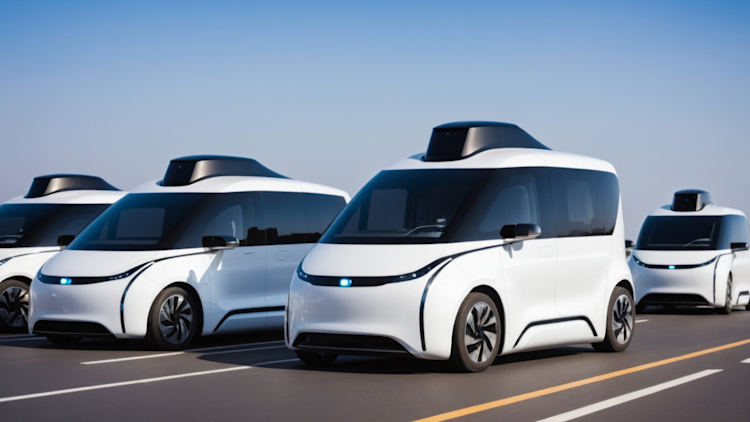
What are Perception Models in Self-Driving Cars?
Perception models use a combination of sensors, cameras, and AI algorithms to interpret data from a vehicle’s surroundings. They identify objects such as vehicles, pedestrians, road signs, and lane markings, determining their location, speed, and movement patterns.
Perception models are fundamental to the success of autonomous car technology, like Waymo for example, enabling them to interpret and understand their surroundings accurately.
These models help with things like obstacle detection, identification of road signs, and navigation through dense or challenging traffic scenarios, all in real time. In cities where road conditions can change quickly, perception models ensure AVs can operate safely and efficiently.
Let’s explore how these models work and their impact on autonomous navigation in complex environments.
Key Components of Perception Models
Computer Vision
Computer vision is a critical component of perception models, enabling robotaxis to “see” their environment through cameras. This technology helps identify objects, detect traffic signals, recognize road signs, and determine lane boundaries. In complex urban areas, computer vision allows robotaxis to make sense of intersections, crosswalks, and even read temporary construction signs, ensuring that the vehicle can respond to changing conditions.
Lidar and Radar Sensors
Lidar and radar sensors provide a detailed 3D map of the vehicle’s surroundings, helping robotaxis accurately detect the distance between objects and their speed. Lidar is particularly effective at mapping the environment, as it can create high-resolution models even in low-light conditions. Radar, on the other hand, is useful for detecting objects at greater distances, such as vehicles approaching at high speeds. Together, these sensors give perception models the spatial awareness needed to navigate safely.
Deep Learning Algorithms
Deep learning algorithms process the vast amount of data collected by sensors and cameras, allowing the vehicle to understand complex patterns. These algorithms are trained on large datasets, enabling the perception model to recognize subtle details like a cyclist approaching from the side or a pedestrian about to step into the road. This ability to predict the behavior of nearby objects is crucial for safe navigation in dynamic urban environments.
How do Perception Models Handle Complex Environments?
Navigating Busy Intersections
One of the most challenging scenarios for robotaxis is navigating through busy intersections. Perception models help by analyzing the movement of other vehicles, pedestrians, and cyclists, allowing the robotaxi to determine the safest moment to proceed. In cities where intersections are packed with activity, these models ensure the vehicle can anticipate changes and react in real-time.
Adapting to Unpredictable Obstacles
Urban environments are full of unexpected obstacles, such as parked cars blocking part of a lane or road work that narrows a street. Perception models enable robotaxis to recognize these obstacles and adjust their route accordingly. This is especially important in areas where road conditions can change from one moment to the next, ensuring that the vehicle can continue on a safe path without needing human intervention.
Understanding Traffic Flow and Patterns
Perception models also help robotaxis by analyzing traffic flow and patterns. For example, they can detect when a lane is slowing down due to congestion and adjust the vehicle’s speed accordingly. They can also recognize when a traffic light is about to change or if a driver is making an unexpected turn, helping the vehicle maintain a safe distance and avoid potential collisions.
Some Challenges in Perception Model Development
- Complexity of Urban Environments Urban areas present a unique challenge for perception models due to the sheer variety of objects and situations they must process. From jaywalking pedestrians to unpredictable traffic patterns, the ability to interpret and respond to these complexities is essential for safe navigation.
- Weather Conditions Weather conditions such as rain, snow, or fog can interfere with the sensors used in perception models. Developing systems that maintain accuracy in all conditions is an ongoing challenge for developers of autonomous driving technology.
The Future of Perception Models in Autonomous Driving
As perception models continue to advance, the capabilities of robotaxis will improve, allowing them to operate in an even wider range of environments. Enhanced AI algorithms, combined with advancements in sensor technology, will enable robotaxis to become more efficient and reliable. These improvements will be crucial for expanding the use of autonomous vehicles in dense urban areas, where safety and adaptability are paramount.
Most existing image datasets are outdated and not relevant to your target location, causing even the most innovative and advanced navigation platforms to perform poorly. See how consistent, quality, up-to-date map imagery can help you build reliable maps, optimize computer vision algorithms, and improve navigation. Discover Bee Map's high-resolution street-level images can enhance your digital image processing projects.
Share Post